AI @ Thomson Reuters
Events: See the Labs Work in Action!
Our team regularly participates in industry conferences, seminars, and workshops. We also host events with AI ecosystem leaders and customer audiences. Check out our upcoming activity below.
Useful links
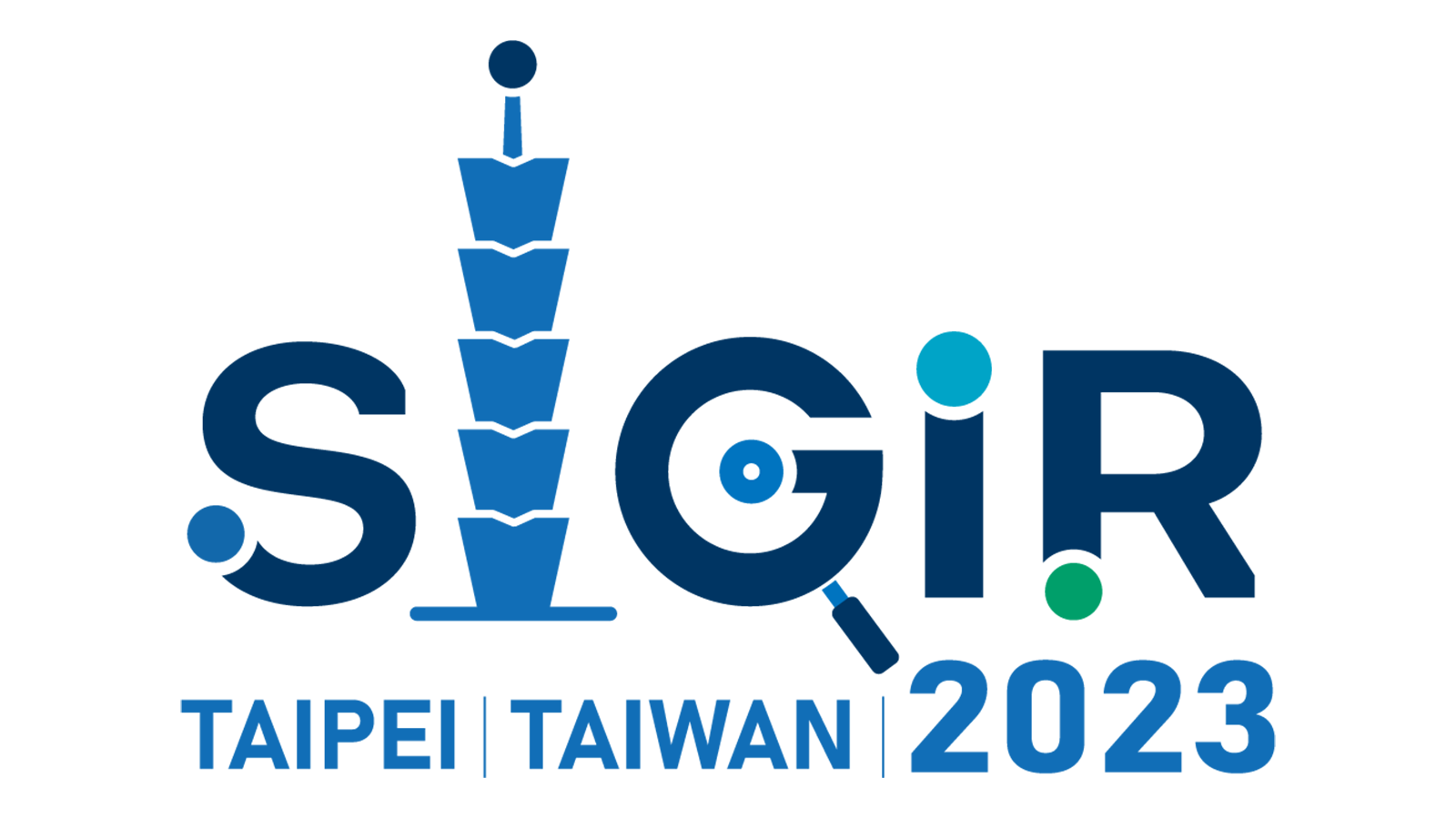
July 23-27, 2023
The 46th International ACM SIGIR Conference on Research and Development in Information Retrieval
Date: July 23-27, 2023
Location: Taipei, Taiwan (Hybrid Conference)
Event Details: View Here
Abstract
SIGIR is the premier international forum for the presentation of new research results and for the demonstration of new systems and techniques in information retrieval.
The conference consists of five days of full papers, short papers, demonstrations, tutorials and workshops focused on research and development in the area of information retrieval, as well as an industry track and social events.
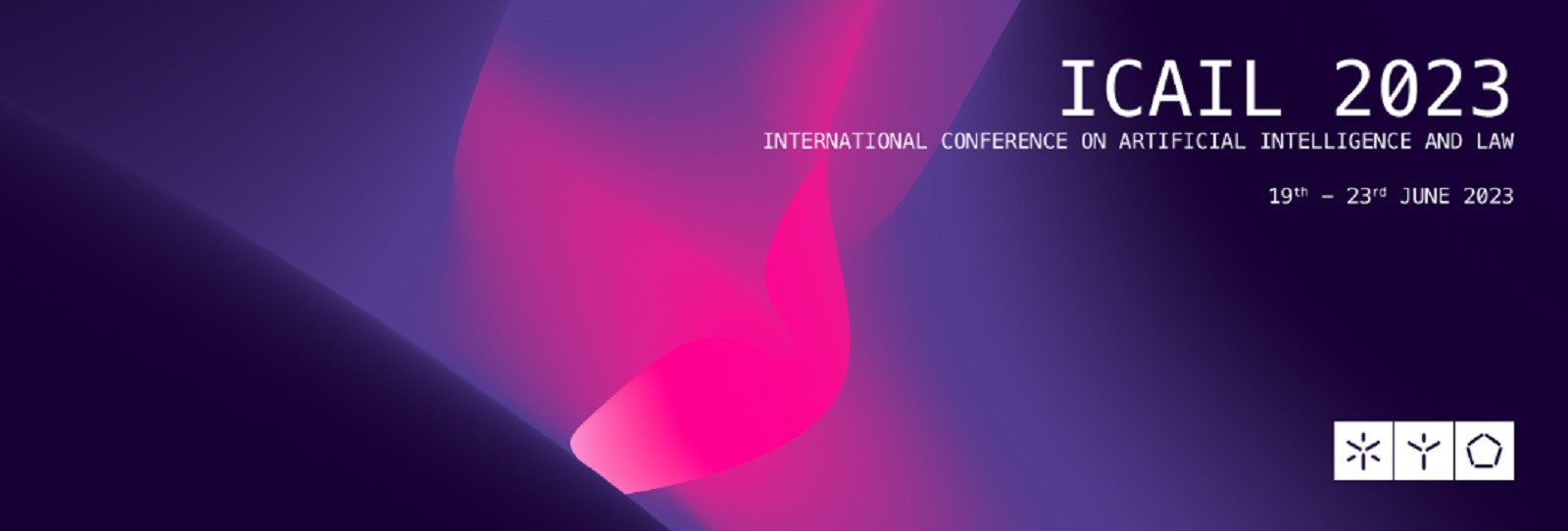
June 19-23, 2023
19th International Conference on Artificial Intelligence and Law - ICAIL 2023
Date: June 19-23, 2023
Location: Braga, Portugal
Event Details: View Here
Abstract
Since 1987, the International Conference on Artificial Intelligence and Law (ICAIL) has been the foremost international conference addressing research in Artificial Intelligence and Law. It is organized biennially under the auspices of the International Association for Artificial Intelligence and Law (IAAIL), and in cooperation with the Association for the Advancement of Artificial Intelligence (AAAI). The conference proceedings are published by ACM. We invite submissions of papers along a regular and three special tracks, technology demonstrations, as well as proposals for workshops and tutorials.
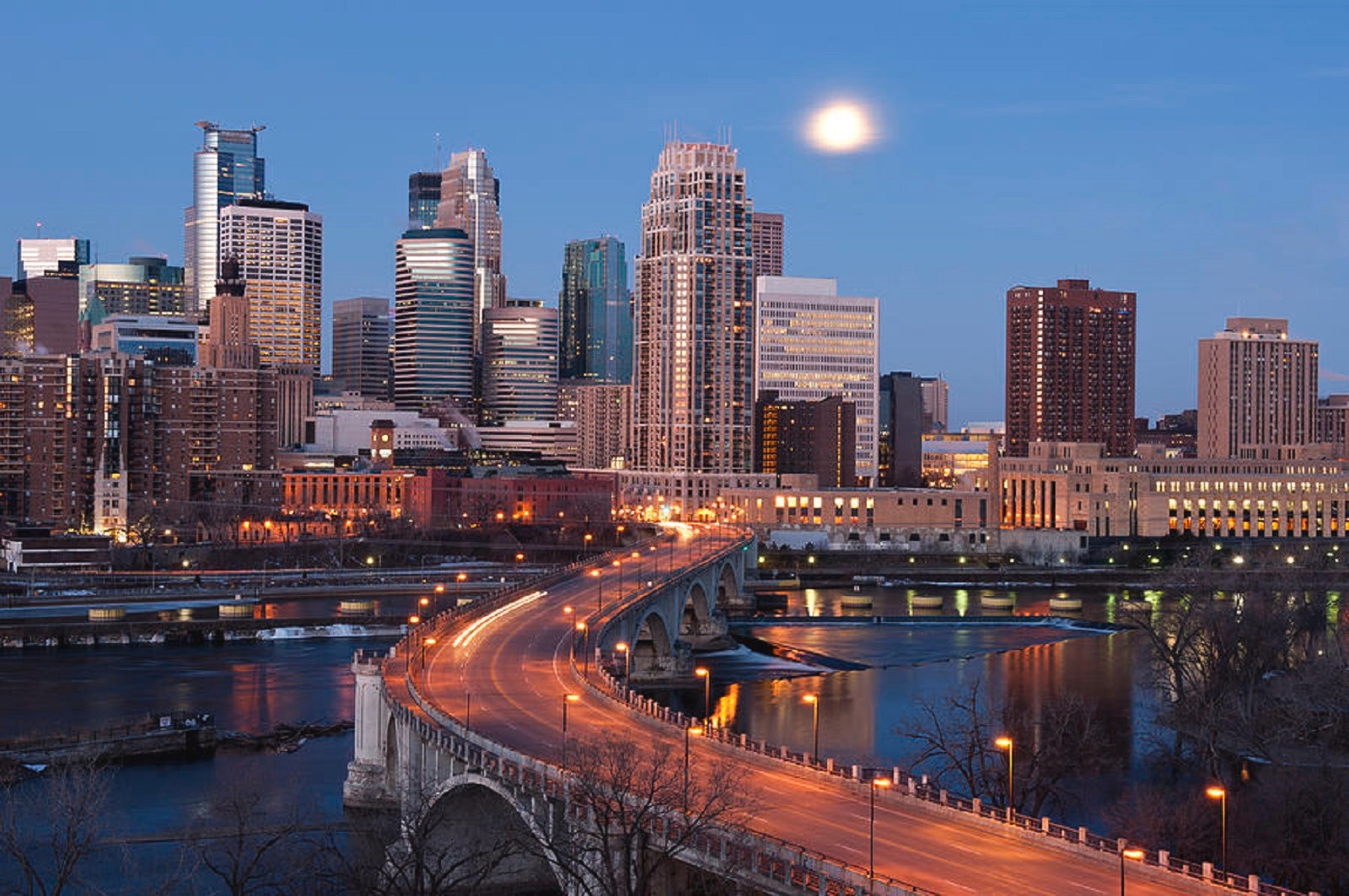
April 27-29, 2023
SIAM International Conference on Data Mining (SDM23)
Date: April 27-29, 2023
Location: Minneapolis, Minnesota (U.S.)
Abstract
Data mining is the computational process for discovering valuable knowledge from data – the core of Data Science. It has enormous application in numerous fields, including science, engineering, healthcare, business, and medicine. Typical datasets in these fields are large, complex, and often noisy. Extracting knowledge from these datasets requires the use of sophisticated, high-performance, and principled analysis techniques and algorithms, which are based on sound theoretical and statistical foundations. These techniques in turn require implementations on high performance computational infrastructure that are carefully tuned for performance. Powerful visualization technologies along with effective user interfaces are also essential to make data mining tools appealing to researchers, analysts, data scientists and application developers from different disciplines, as well as usable by stakeholders.
The SDM conference provides a venue for researchers who are addressing these problems to present their work in a peer-reviewed forum. It also provides an ideal setting for graduate students to network and get feedback for their work (as part of the doctoral forum). Everyone new to the field can also learn about cutting-edge research by hearing outstanding invited speakers and attending presentations and tutorials (included with conference registration). A set of focused workshops is also held on the last day of the conference. The proceedings of the conference are published in archival form and are also made available online.
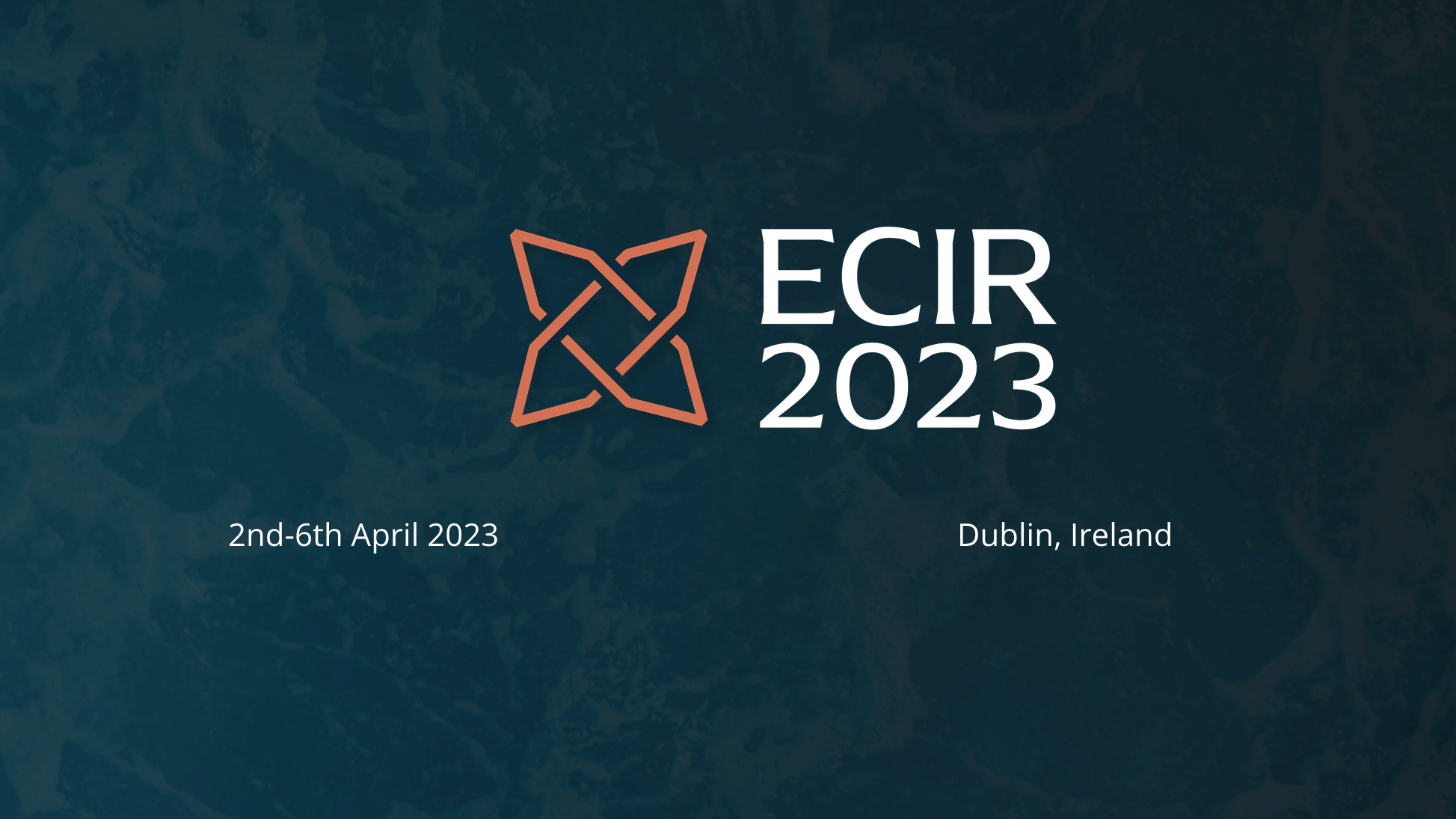
April 2-6, 2023
Legal Information Retrieval (Legal IR) Workshop at ECIR 2023
Date: April 2-6, 2023
Location: Dublin, Ireland
Event Details: View Here
Abstract
The 45th European Conference on Information Retrieval (ECIR) is the annual premier European forum for the presentation of new research results in the broadly conceived area of Information Retrieval. ECIR provides an opportunity for both young and established researchers to present research papers reporting new, unpublished, and innovative research results. ECIR has traditionally had a strong student focus and papers whose sole or main author is a postgraduate student or postdoctoral researcher are especially welcome. ECIR 2023 is planned to be held as a physical conference (with support for remote attendance) from the 2nd to the 6th of April 2023 in Dublin, Ireland.
Previous events
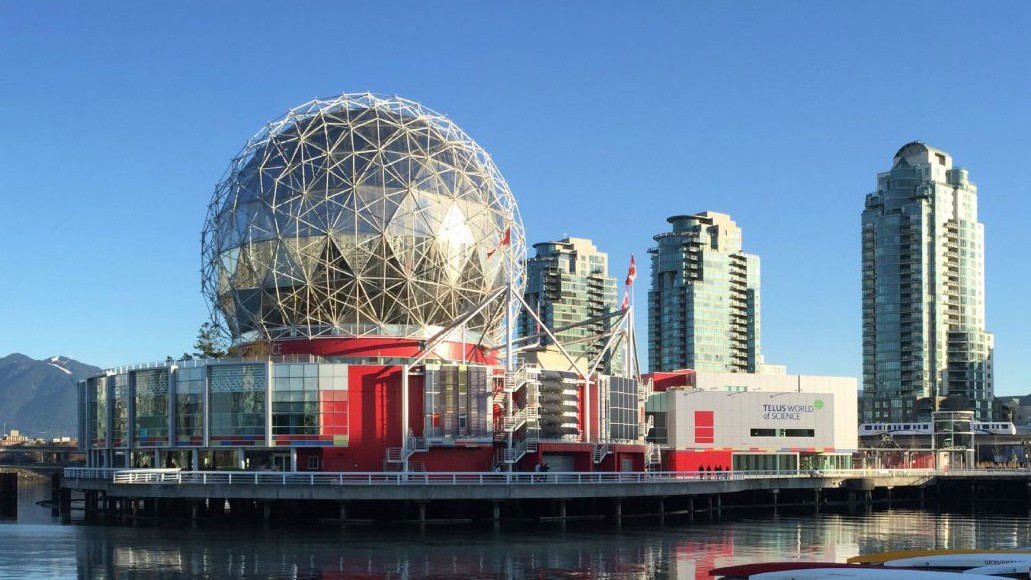
February 22-March 1, 2022
AAAI conference series
Date: February 22-March 1, 2022
Location: Vancouver, BC, Canada
Event Details: View Here
Abstract
The purpose of the AAAI conference series is to promote research in artificial intelligence (AI) and foster scientific exchange between researchers, practitioners, scientists, students, and engineers in AI and its affiliated disciplines. AAAI-22 is the Thirty-Sixth AAAI Conference on Artificial Intelligence. Like previous AAAI conferences it will feature technical paper presentations, invited speakers, workshops, tutorials, poster sessions, senior member presentations, competitions, and exhibit programs, all selected according to the highest standards. AAAI-22 will also include additional programs for students and young researchers.
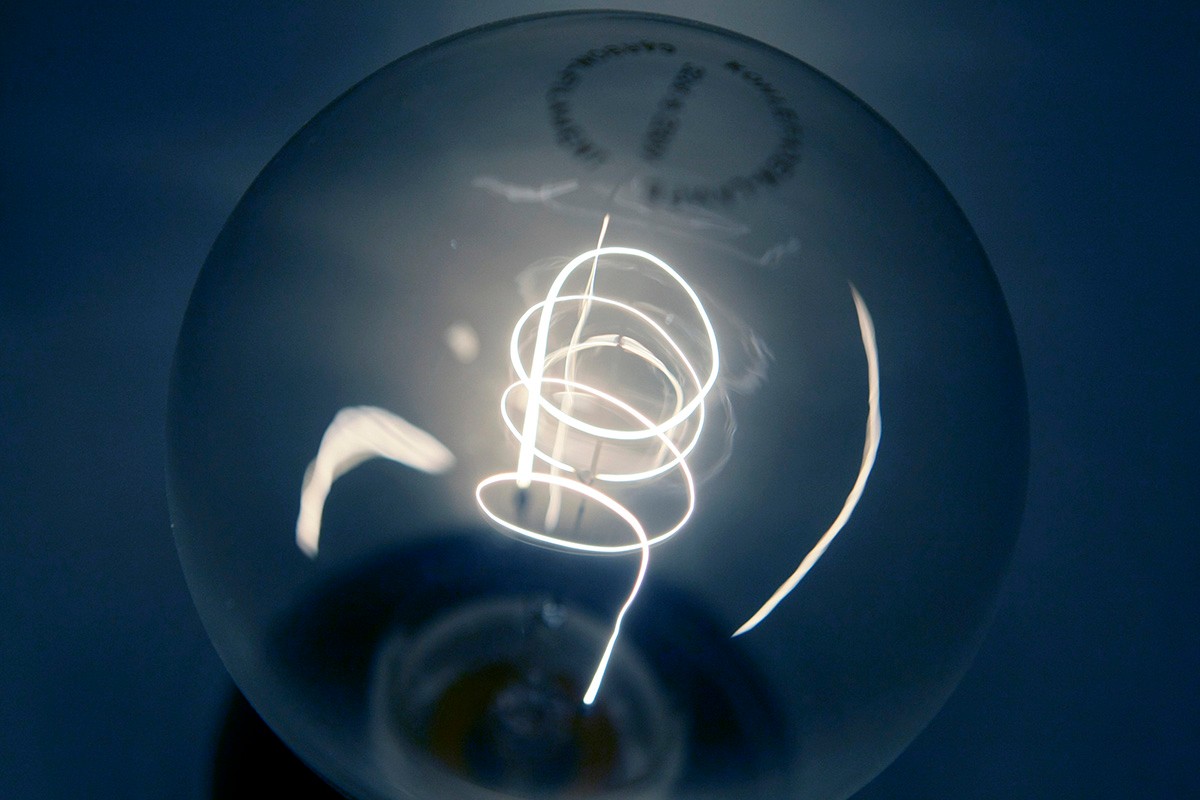
December 7-10, 2021
Mining and Learning in the Legal Domain
(MLLD2021)
Date: December 7-10, 2021
Location: Auckland, New Zealand
Event Details: View Here
Abstract
In conjunction with the 21st IEEE International Conference on Data Mining, December 7-10, 2021, Auckland, New Zealand
The increasing accessibility of large legal corpora and databases create opportunities to develop data driven techniques as well as more advanced tools that can facilitate multiple tasks of researchers and practitioners in the legal domain. While recent advancements in the areas of data mining and machine learning have gained many applications in domains such as biomedical, healthcare and finance, there is still a noticeable gap in how much the state-of-the-art techniques are being incorporated in the legal domain. Achieving this goal entails building a multi-disciplinary community that can benefit from the competencies of both law and computer science experts. The goal of this workshop is to bring the researchers and practitioners of both disciplines together and provide an opportunity to share the latest novel research findings and innovative approaches in employing data analytics and machine learning in the legal domain.
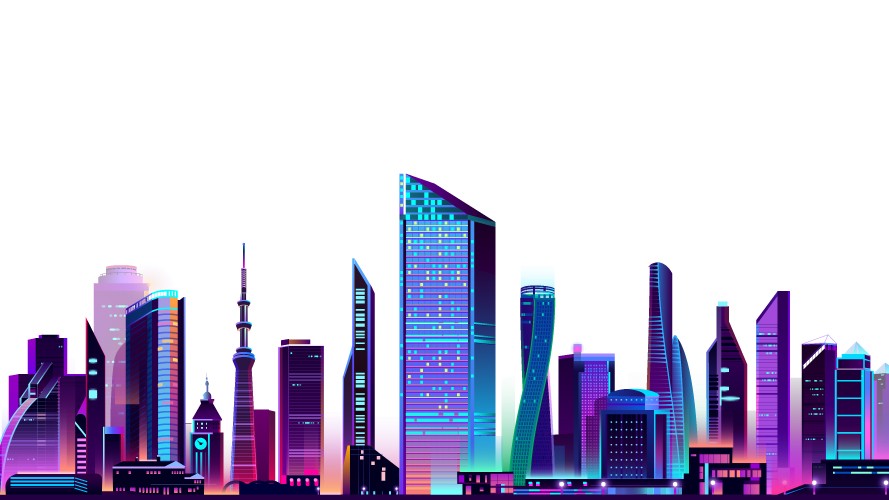
October 14-15, 2021
Data Innovation Summit 2021
Date: October 14-15, 2021
Location: Kistamässan, Stockholm
Event Details: View Here
Abstract
Data Innovation Summit is constructed so it equally addresses all the elements of data-driven and AI-ready business: data, people, processes and technology. The event is built to be both business and technical, practical and inspirational, realistic and futuristic, educational and exciting, regional and global, live and digital, general and niched, inspiring and influential.
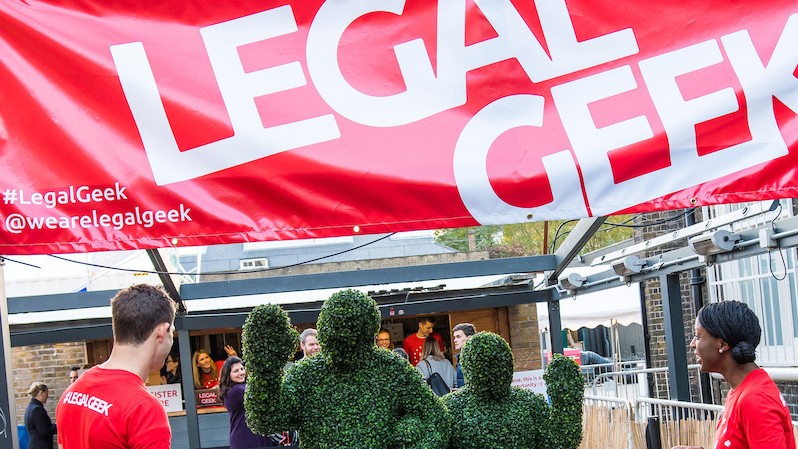
October 5-6, 2021
Legal Geek Conference
Date: October 5-6, 2021
Location: London, England
Event Details: View Here
Abstract
The Legal Geek Conference is back! We are bringing together law firm leaders, general counsel, investors, scaleups, policymakers, startups and innovation-fanatics for two days of inspiration and knowledge-sharing. We’re bringing the community back together. Check out the Labs in the speaker sessions, exhibition area and workshops.
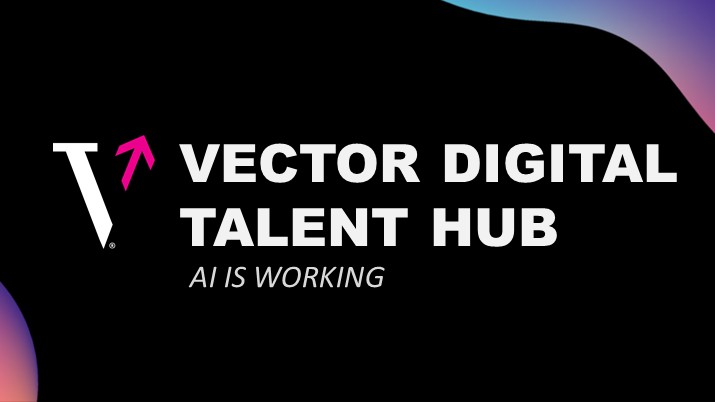
September 24, 2021
AI Summit and Career Fair 2021
Date: September 24, 2021
Location: Virtual
Abstract
Vector’s annual AI Summit & Career Fair (held virtually) provides an opportunity for AI graduate students, researchers & alumni* to hear from industry leaders, practitioners, and researchers in AI. Explore career opportunities at organizations leading AI research and adoption, learn about what it takes to get hired as a recent graduate, and network with students and alumni across the province.
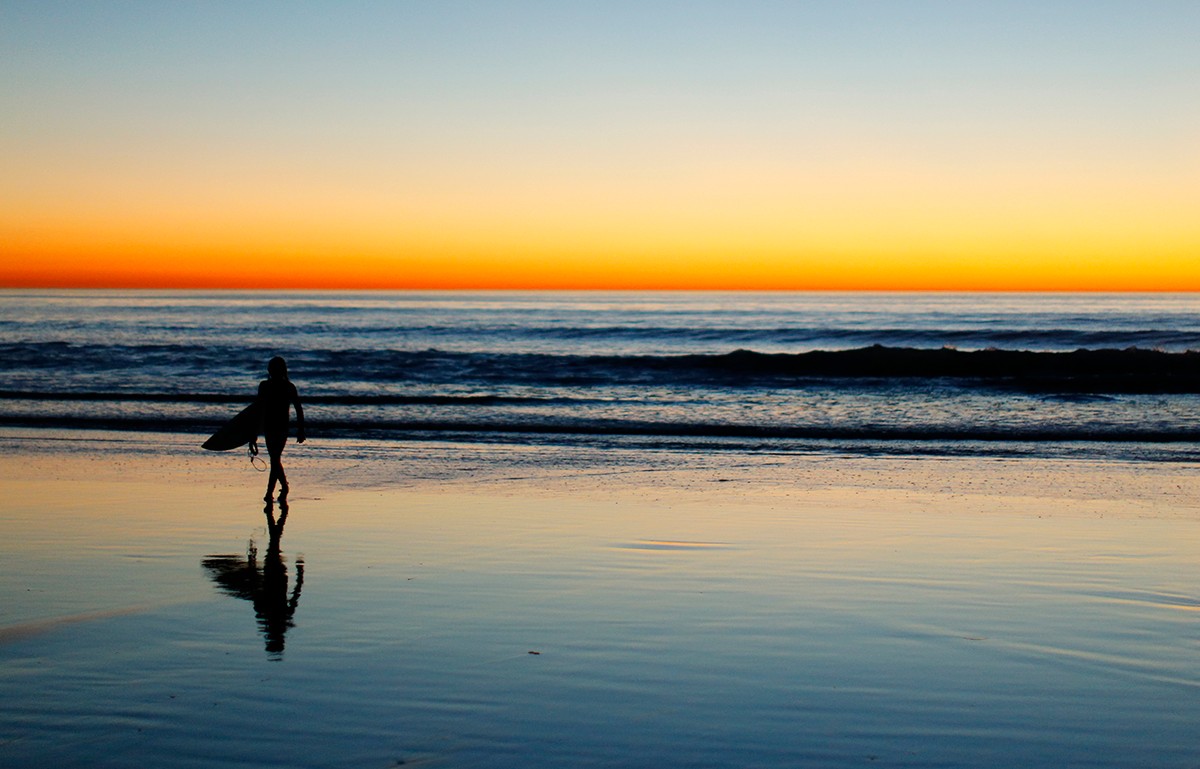
June 17, 2021
AI Ethics: It’s Time
Date: June 17, 2021
Location: Virtual
Abstract
As Artificial Intelligence (AI) and autonomous decision-making systems take hold and rapidly develop, how can Canada ensure this powerful technology upholds our most important values? Fairness, inclusion, democracy, individual privacy, economic security, public safety and sustainability. Ethical AI: It's time — brought to you by Thomson Reuters; Communitech, the champions of Tech for Good®; and CityAge — brings together some of the top minds in AI, business and society to drive conversation around how we can ensure this transformative and rapidly evolving technology benefits everyone. We have a duty in front of us to leverage these technologies to solve some of the world’s most pressing challenges, but we must do so safely.
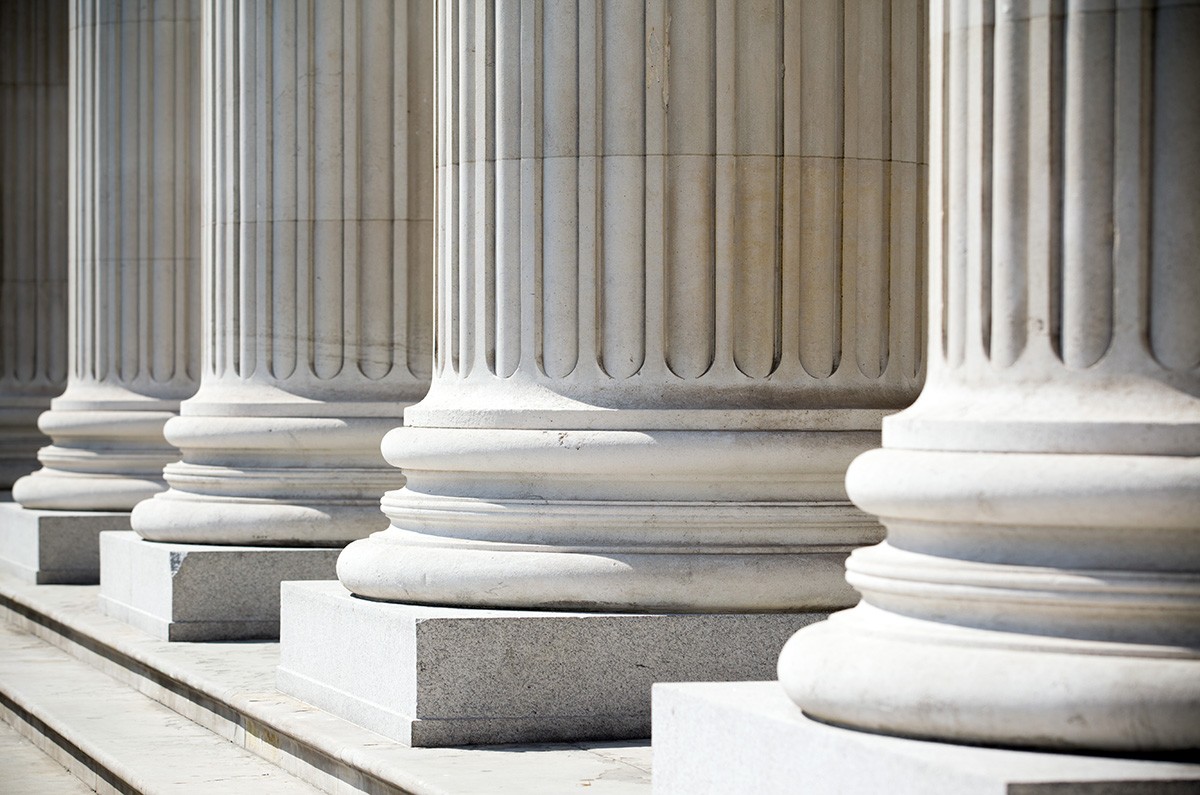
May 31-June 04, 2021
Symposium on AI and Law
Date: May 31-June 04, 2021
Location: Virtual
Event Details: View Here
Abstract
The Symposium on Artificial Intelligence and Law (SAIL) is a five-day, virtual event that aims to bring together experts from Industry and Academia to discuss the future of AI and Law. The Symposium aims to provide a venue for academic and industrial/governmental AI-Law researchers and law professionals to come together, present and discuss research results, use cases, innovative ideas, challenges, and opportunities that arise from applications of AI in the Legal Domain. The symposium is also meant to foster collaborations between the Legal and the Artificial Intelligence, Data Mining, Information Retrieval, Natural Language Processing, and Machine Learning communities.
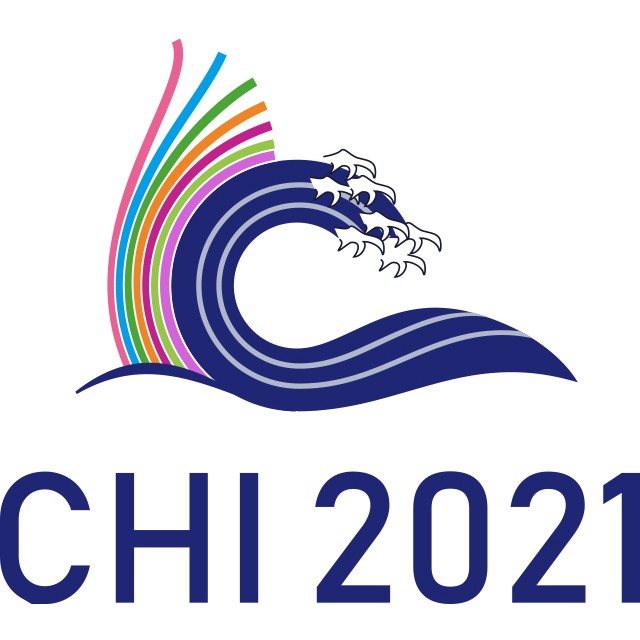
May 11, 2021
CHI 2021: Towards Explainable AI
Assessing the Usefulness and Impact of Added Explainability Features in Legal Document Summarization
Date: May 11, 2021
Location: Virtual
Event Details: View Here
Abstract
This study tested two different approaches for adding an explainability feature to the implementation of a legal text summarization solution based on a Deep Learning (DL) model. Both approaches aimed to show the reviewers where the summary originated from by highlighting portions of the source text document. The participants had to review summaries generated by the DL model with two different types of text highlights and with no highlights at all. The study found that participants were significantly faster in completing the task with highlights based on attention scores from the DL model, but not with highlights based on a source attribution method, a model-agnostic formula that compares the source text and summary to identify overlapping language. The participants also reported increased trust in the DL model and expressed a preference for the attention highlights over the other type of highlights. This is because the attention highlights had more use cases, for example, the participants were able to use them to enrich the machine-generated summary. The findings of this study provide insights into the benefits and the challenges of selecting suitable mechanisms to provide explainability for DL models in the summarization task.
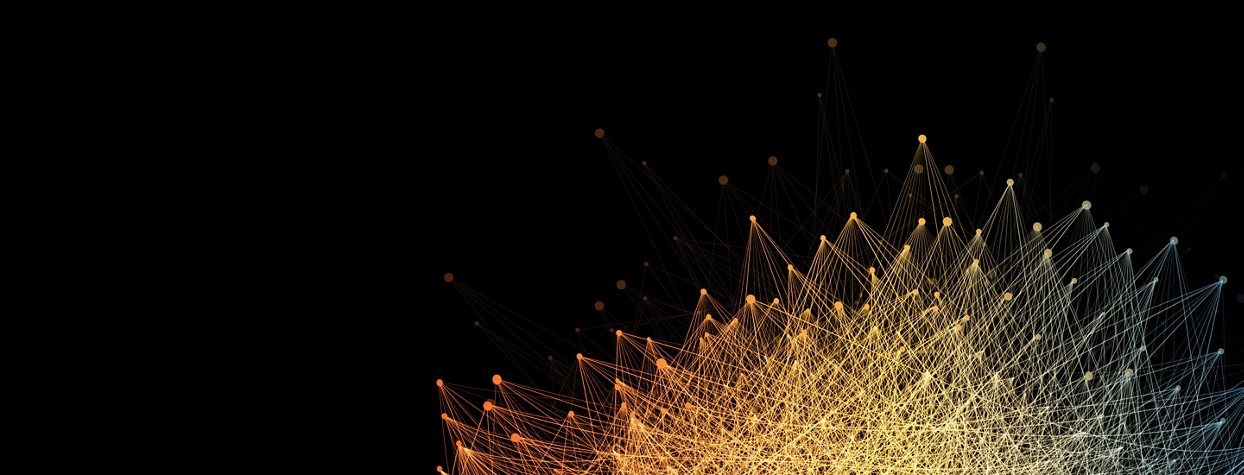
AI @ Thomson Reuters
Thomson Reuters and Generative AI: Defining a new era for how legal and tax professionals work