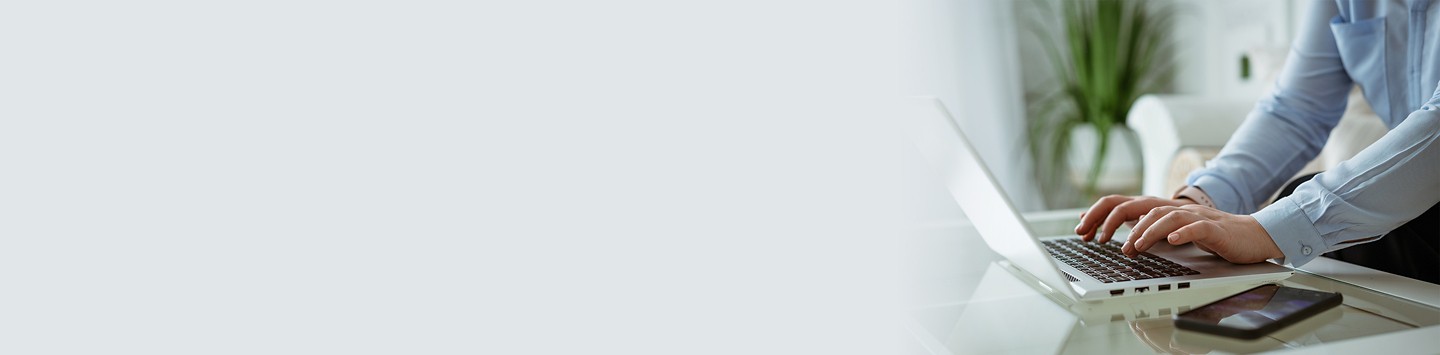
Artificial intelligence
Human-Centric AI at Thomson Reuters
Trust is at the core of everything we do at Thomson Reuters, including the design, development, and deployment of AI systems. In the spirit of our Trust Principles we need to ensure that we are also the provider of trustworthy AI solutions. AI and its use are currently largely unregulated and globally adopted standards and principles are in development. At Thomson Reuters we are contributing to the progress of these domains, by ensuring we not only strengthen trust in our own AI systems, but that we are supporting the progress of trustworthy AI throughout society.
The Human-Centric AI research theme is a multidisciplinary approach to addressing the challenges AI faces in terms of full adoption and trust. It gains in importance as human workflows are increasingly intertwined with AI systems to support them. It is closely tied to AI Ethics concepts such as interpretability, explainability, transparency, bias/fairness, privacy, security and societal impact, which are central concepts in TR’s AI Principles. We are investigating how to design, build, test and deploy AI systems with a human-centric mindset, and are establishing thought-leadership in this domain in collaboration with internal stakeholders, industry partners, and universities.
The objective of our research in this domain is to demonstrate how we put our AI Principles into practice; maximize the effectiveness of AI features we build and deploy; and to ensure we are making our best efforts to address complex questions under this theme.
Our Work:
Norkute, Milda, Nadja Herger, Leszek Michalak, Andrew Mulder, and Sally Gao. 2021. “Towards Explainable AI: Assessing the Usefulness and Impact of Added Explainability Features in Legal Document Summarization.” In Extended Abstracts of the 2021 CHI Conference on Human Factors in Computing Systems. CHI EA ’21. New York, NY, USA: Association for Computing Machinery.
Schleith, Johannes, Nina Hristozova, Brian Chechmanek, Carolyn Bussey, and Leszek Michalak. 2021. “Noise over Fear of Missing Out.” In Mensch Und Computer 2021 - Workshopband, edited by Carolin Wienrich, Philipp Wintersberger, and Benjamin Weyers. Bonn: Gesellschaft für Informatik e.V.