Can artificial intelligence solve the “fake news” problem?
Researchers at the University of Zurich are trying to find out by using “deep learning” machine intelligence to identify bias in news coverage from across the ideological spectrum.
The project is part of the university’s new Digital Society Initiative, a cross-disciplinary network of academics and scientists who are studying how the digitization of society impacts communication, health, work, community, and democracy. Funded by the Heidelberg Academy of Sciences, the Zurich team is building on research conducted at the University of Wuppertal and the University of Konstanz by Dr. Bela Gipp, an information scientist who is doing similar research on using artificial intelligence to identify academic plagiarism.
Framing & bias
In Zurich, the fake-news project is led by Dr. Karsten Donnay, an assistant professor of Political Behavior and Digital Media at the University of Zurich. His team’s effort to “detect and reveal” biased news coverage is among the first serious attempts to use artificial intelligence to combat the spread of false and misleading information on the internet.
“Framing of the news matters,” Donnay said recently during an AI@TR Invited Speaker Series event presented online and hosted by Thomson Reuters. “It matters how news is covered,” he said, adding that it especially matters that people be able to identify “biased, false, and often sensational information disseminated under the guise of news reporting.”
For example, consider the following two sentences describing the same event:
U.N. arms inspectors said they had withdrawn two U-2 reconnaissance planes over Iraq for safety reasons.
Iraqi fighter jets threatened two American U-2 surveillance planes, forcing them to abort their mission and to return.
In the first sentence, the words withdrawn, reconnaissance, and safety are relatively neutral. In the second sentence, however, the words threatened, surveillance, and forcing have a more menacing tone. Both might be technically correct, but the words convey different sub-textual meanings, so their framing is different.
Teaching the AI
According to Donnay, the project’s ultimate goal is to create a news aggregation website that uses an AI tool to identify bias and deception in the daily news, then sorts and presents stories in an ideologically neutral way that readers can easily understand and will, hopefully, come to trust. The project is still in its early phases, however, and the difficulties are formidable. First, the AI tool needs to learn how to recognize bias, which means it needs to be able to parse nuances in language and context that even careful human readers have trouble distinguishing.
Therein lies one of many challenges.
Programming a computer to identify human bias in writing essentially means teaching it how to read. But reading itself is a complex mental process that scientists don’t fully understand, and artificial intelligence is only as smart as the programming and data that supports it. Nevertheless, Donnay’s team is attempting to “teach” its AI to recognize bias by mimicking the subconscious processes human beings use to evaluate the veracity of information they absorb. Unfortunately, human beings aren’t very good at identifying bias, either; and the whole idea of determining the degree of “truth” in any given news story can lead down several philosophical rabbit holes that science may be ill-equipped to address. If one person’s terrorist is another’s “freedom fighter,” after all, then truth is at least partially a matter of perspective.
As Donnay explained, the difficulty in solving the fake news problem begins with recognizing that the process of gathering, reporting, writing, editing, and disseminating the news is itself imperfect. News organizations have owners and advertisers and target audiences, for example, and these factors influence their selection and presentation of stories. Too, every sentence a writer produces is a series of judgment calls about which words to use and what ideas to emphasize, all of which are a reflection of education, experience, culture, and so on. There is also the perspective of the reader to consider.
It’s (very) complicated
Among the first challenges Donnay and his team are facing is the task of creating a large enough data set of linguistic examples for an AI tool to use as a framework for analysis. Past efforts to create “dictionaries” of words and phrases have not worked particularly well, Donnay noted. Instead, his team is trying to develop a more thorough deep-learning approach, which requires a great deal of annotated data.
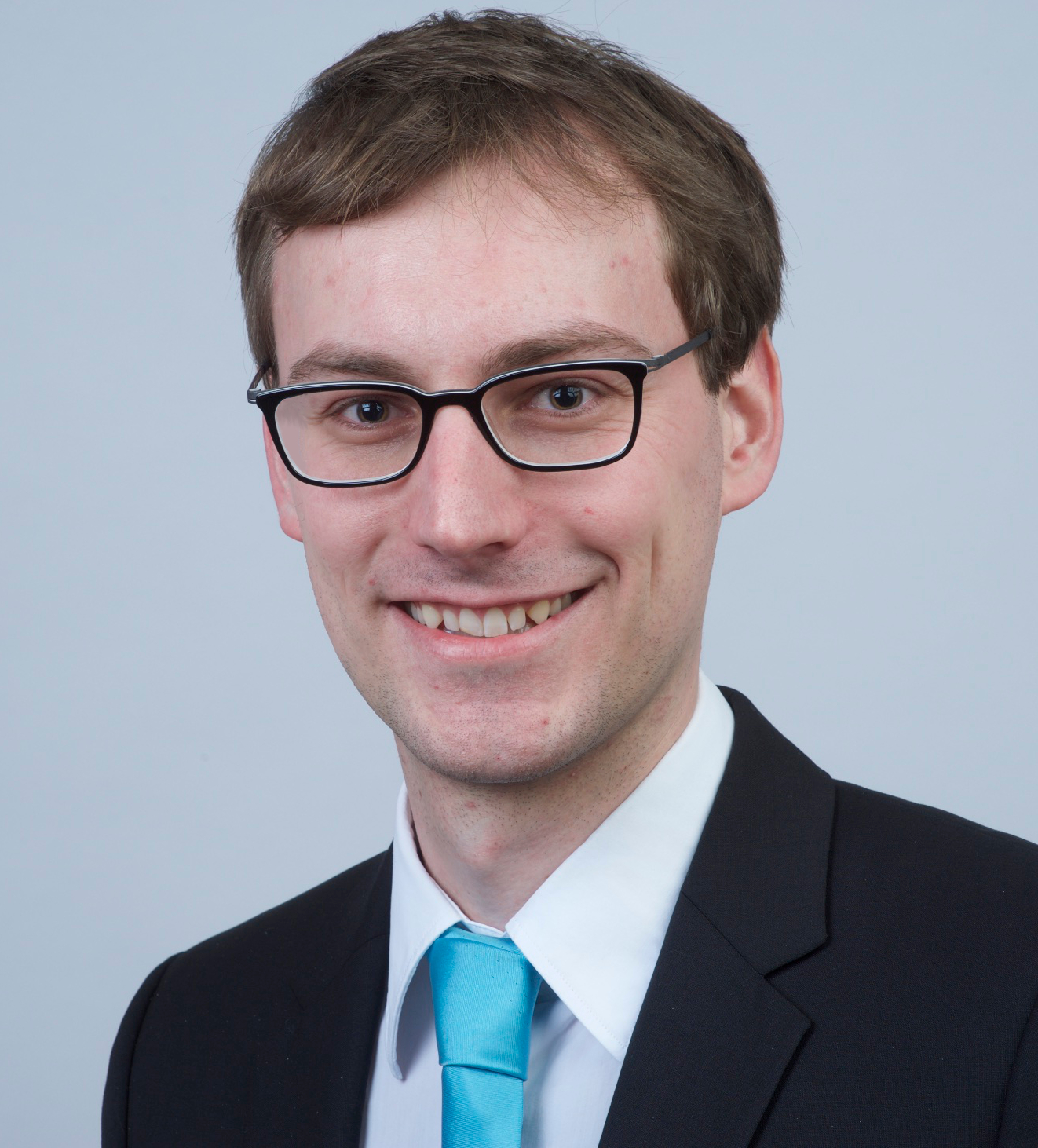
In a pilot project, the team first focused on the simpler task of sentiment analysis to illustrate how this approach can be used to weigh the degree to which ideas in a news story are framed as “positive” or “negative.” The next major challenge was to expand the logic of sentimental analysis to build an annotated database large enough to apply neural modeling techniques that mimic how the human brain deduces more complex word meanings and intent.
A team of hundreds of coders analyzed and scored tens of thousands of sentences to create a new benchmark data set for detecting media bias. Once the AI tool “learns” enough examples to identify bias issues at the level of word choice and sentence structure, it can expand its analysis to the larger context of paragraphs and entire stories.
However, in order to analyze a news story for bias and compare it with other stories on the same subject, the AI tool needs to be able to recognize that the core subjects and concepts discussed in multiple stories are connected. “For this, there are no out-of-the-box tools that work really well, which is why we came up with our own approach,” Donnay explained. Developed by the Dr. Gipp’s team, that approach involves using a six-step merging heuristic to identify subjects and concepts central to each story, as well as other signifiers such as contextual clues and verb choices.
“We realize this has to be done in steps,” Donnay added. And the next step — enlarging the AI tool’s capabilities to identify false and misleading information, or “fake news” (rather than simply biased news) — is a big one.
Are news sources trustworthy or not?
According to Donnay, “fake news is structurally different” from the framing involved in determining bias, because identifying fakery involves distinguishing between “trustworthy” and “untrustworthy” news sources, as well as facts, lies, rumors, and innuendo. “Determining the veracity of news is very hard,” Donnay conceded, adding that the framing of news stories itself creates inherent biases, and understanding this is a first important step towards more balanced and trustworthy news.
Donnay said he hopes to develop a prototype website sometime next year, but there is still plenty to be done. For starters, the data sets required for accurate neural modeling need to be significantly enlarged; a broad sampling of news across domains and sources needs to be coded; and the quality of the coding, which is done by humans, needs to remain consistently high.
Too, even if the news aggregation website Donnay envisions is eventually operational, there is no guarantee that people will trust its judgment. The site would also be more useful as a global resource if it worked in languages other than English, but that too is a challenge for another day.
“In 2021, once everything is finished, we plan to run a large-scale experiment using the final tool,” Donnay said. That’s the “social science” part of the experiment, he explained — the part in which readers react to AI-selected content and report whether they can tell a difference in how the facts are presented.
Locating “the truth” about news may be too much to ask, of course, but any progress toward a basic agreement of facts would be a step in the right direction.