Legal organizations and others that depend on artificial intelligence to power their data analytics and decision-making need to ensure they are addressing potential bias in data collection
No artificial intelligence (AI) or machine learning algorithm is developed in a vacuum. Just like any piece of technology, or any corporate process for that matter, AI is typically developed with a specific goal in mind.
At times, however, this blind focus on achieving that singular objective could actually lead to a mismanaged AI process that doesn’t take potential biases into account, researchers say, adding that these biases could have been baked in the AI all the way back at the data collection stage. As a result, the idea of instituting fairness metrics into AI development is starting to gain popularity in the tech community — not only for ethical and social reasons, but to ensure a more complete end product emerges from the AI processes.
The idea of fairness within AI comes from the idea that not all data is created equal, whether it’s measuring human populations or words in a legal document. If an AI algorithm is measuring the potential risk within a procurement contract, for example, the context matters, whether that contract is procuring coffee mugs or nuclear material. If it’s measuring whether a public economic policy is being applied equally across different races, it matters whether the population data is New York City or the rural Midwest. Fairness in AI means planning for these differences in data to make the end result representative of the goal that developers are actually trying to have the AI process tackle.
Sometimes, however, modern cost and time considerations can get in the way of fairness, says Cao (Danica) Xiao, vice president of machine learning and Natural language processing (NLP) at software company Relativity. Xiao came into the legal industry recently, but she previously spent time as an AI research leader in the healthcare and technology fields. When discussing what fairness means when it comes to legal AI development, Xiao draws a parallel to a healthcare development with which many are now familiar: the COVID-19 vaccine.
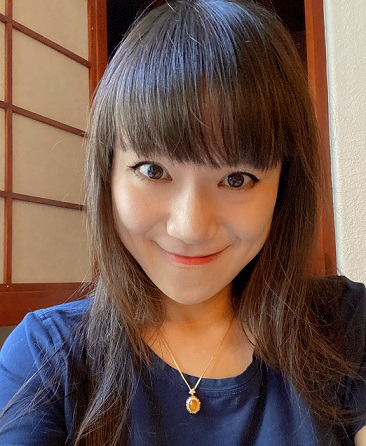
A December 2020 study from MIT revealed that despite the COVID-19 vaccine efficacy figures touted by providers Pfizer and Moderna, the true efficacy of the vaccines varied highly by race. The study measured the number of people whose cellular immune system was not predicted to robustly respond to the vaccine; and those figures varied wildly by race, from less than 0.5% of white clinical trial participants without a robust response up to nearly 10% of Asian participants.
The issue, Xiao says, is one of initial sampling. White populations tend to be overrepresented in vaccine and drug clinical trials due to a number of factors, including education and income level, proximity to news promoting the availability of trials, and sheer population size in the US. But many clinical trials, particularly for a vaccine as time-sensitive as COVID-19, tend to have one constraint that rules over all: the time it takes to recruit trial participants.
“So if we only want to minimize time, then the majority of cases, the majority of patients and people we recruit to the trial, they represent the majority group of the population,” Xiao explains. “That’s a fact that we cannot avoid.” As a result, the trial’s results will be skewed towards that overrepresented group.
Awareness of those differences can go a long way, however, whether in developing healthcare trials or creating representative data samples to run against an AI algorithm. That’s why, while Xiao concedes that she’s heard the legal industry is largely behind the healthcare industry in its adoption of AI technologies, she’s more interested in changing how legal organizations approach artificial intelligence before ever running a single algorithm.
Tools to lessen AI bias
Drawing from AI development in other industries, there are a number of fairness metrics that those data scientists exploring legal AI can take into account up front. A simple one is identifying subgroups early on to make sure there are representative populations of each type, be it demographic-centric subgroups such as race or gender, or contextual subgroups such as various types of matters across a firm.
A slightly more complex metric that Xiao points to is known as privacy-preserving federated learning — the idea that researchers should consider data sets from multiple locations, lessening the bias that occurs in each individual data set by combining them in a federated manner. “We train a local model from each location, but we don’t use the local model to represent the total behavior,” Xiao says. “We train a global model on top of the local model, and we will adjust the parameter of the model to make sure the final global model will consider each different heterogeneous pattern, and the web will be equally good for different populations.”
Fairness in AI means planning for differences in data to make the end result representative of the goal that developers are actually trying to have the AI process tackle. Sometime, however, modern cost and time considerations can get in the way of fairness.
Using data science techniques, there are also ways to amplify rare outcomes, which are crucial to find in healthcare and law alike. Or put a different way, if the purpose of a particular AI algorithm is to find a needle in a haystack, it’s important for the needle to stick out rather than be dismissed as noise. These rarities can also identify anomalies that are important to investigate further. The goal of rarities detection is “to amplify the pattern in those rare samples to amplify their voice, to boost their patterns, to make sure our final model will be able to capture those patterns and will learn the patterns in those data,” Xiao notes.
For legal organizations dipping their toes into AI for the first time, perhaps the most straightforward way to lessen bias in AI models is to make sure data sets are up to date. For example, if you are looking at a natural language processing test that links women to their profession and the training data comes from 20 or 30 years ago, professional titles for women may look a lot more different than they do today.
This is not only a question of fairness, Xiao says, but one of correct outputs. “If we only test the model against the old data, we might see lower accuracy,” she adds. “We need to consider those new trends and consider those new advancements and all those inclusion metrics in the model, and the model will be more and more accurate moving forward on the future data.”
As AI models become relied upon for more and more legal and professional work, particularly as technology’s capabilities for analyzing data and making predictions continue to grow, it’s crucial for the legal industry to adopt fairness methodology now and develop fairness metrics into AI development early.
“When we make a prediction, we need to consider this advanced context information to make sure the prediction is more accurate, but it will be a long process,” Xiao says. “We need to continue improving the solution.”