Jan 27, 2023 | Our Purpose
The road to building trust with AI systems
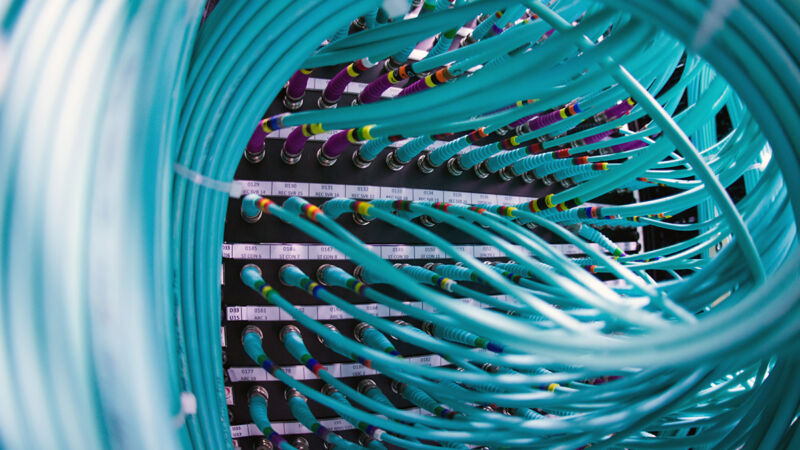
Milda Norkute, Lead Designer, Thomson Reuters Labs
Thanks to recent advances in artificial intelligence (AI) and machine learning (ML) such as the ability to generate language that aligns with human speaking and writing patterns, AI is no longer the future – it’s here.
AI solutions are increasingly integrated into many technology solutions across various sectors: in our cars, work tools and even our pockets. AI methods are achieving unprecedented performance levels when solving increasingly complex computational tasks, making them more important to the future of our society than ever before.
However, as decisions made by AI systems start having real impact on our lives – for example, in deciding whether someone should be approved for a mortgage or not – an important question has emerged: What level of trust can (and should) we place in these AI systems?
Understanding the AI trust gap
To trust AI decisions, people need to know how an AI system arrives at its conclusions and recommendations. Understanding how a system works gives us a sense of predictability and control. This is because humans are driven to acquire and provide explanations. Within months of uttering their first words, children ask, “Why?” Explanations provide us with a sense of understanding, and how things work has been essential to our survival as a species. Furthermore, being able to communicate why we do things is crucial for communication and cooperation with one another.
Since explanations are essential for enabling collaboration between humans, they are also important for humans who must rely on systems powered by AI. After all, it takes many years, work and training together side-by-side for human teams to trust each other unconditionally and anticipate each other’s actions without any explanation. It is even harder to trust AI systems that we do not know and understand so well.
However, larger, more powerful and therefore more useful AI systems (also known as deep neural networks) unfortunately often cannot offer explanations to users interacting with them. They are essentially a ‘black box.’ Researchers and users typically know their inputs and outputs, but it is hard to see what’s going on inside and we don’t know how all the individual neurons work together to arrive at the final output. As an example, this means that we cannot know how exactly GPT-3 wrote an email or a story.
The role of explainability in AI systems
To overcome this issue, companies selling AI solutions have increasingly looked for ways to offer some insight into how such black box AI algorithms reach decisions. They primarily focus on developing additional algorithms which approximate the behavior of a ‘black-box’ system to offer post-hoc interpretations of the original AI decision.
For example, AI engineers may build an AI system which decides whether documents should be classified as confidential or not. In addition to the decision of the system about a document, they present users with a set of words that an additional algorithm, seeking to ‘explain’ the decision of the AI system, identified as important for the decision and the different prediction probabilities for the two possible prediction outcomes.
However, in studies where such explanations were tested with the users, they were often not sure what the numbers meant – for example, 50% probability seemed high to one user, but not another. The room left for interpretation in such explanations can make it difficult for the users to rely on them and introduce doubt into AI suggestions themselves. Therefore, increasing trust through such explainability approaches does not always yield results.
To achieve true explainability, AI practitioners should look for ways to explain the AI solutions they are developing right at the start of the development process of these algorithms rather than as an afterthought. More efforts should be devoted to understanding what happens inside the ‘black box’ in addition to focusing on reducing computational costs, time and creating better outputs when improving AI models.
Additionally, AI practitioners should put the user at the center when developing new technology and understand their explainability needs. If transparency is strongly desired by the users in some cases, in order to have more transparency, simpler, less powerful models may have to be used, despite how tempting playing with the newest technology may seem.
The road to AI trust built by humans
The only true constant so far in our world has been change and AI is just an additional new piece to the puzzle we are all putting together. We each have a piece to create and place in the puzzle. Technologists must do their part in creating AI systems that humans can trust by putting greater emphasis on explainability and transparency when building such systems.
Governments must do more in providing guidelines and regulations for the industry, to foresee and prevent potential misuse of data and AI. For example, the introduction of “right to explanation” in GDPR in 2016 is a step in the right direction. Companies need to be more transparent to the general public about how our data is supporting the development of better AI solutions and products.
Nevertheless, the challenges that lie ahead in achieving the full potential of AI and incorporating it into our lives are truly exciting to work on for many in the field, including our AI teams at Thomson Reuters. With incremental innovation combined with human values and intelligence, we are working towards positive breakthroughs in combining AI with human expertise in critical areas such as legal, news, government, and many others – this is just one of the ways we’re helping inform the way forward at Thomson Reuters.